A SaaC is both a novel instructional design concept and an agile deployment of data solutions, it delivers two things:
-
A working solution to a real data problem that real people experience in their specific business function
-
A sustainable Data Science education centered around creating this specific solution
SaaC is not just a new acronym, the approach will be increasingly used as it solves what is not working today:
decades of abysmal skill gaps in regards to data education,
data skills shortage,
errors and lack of control due to our addiction to and relying only on “black box” AI systems, …
For a course to deserve this name, there is a set of strict requirements: how it should be conceived, what data problem does it solve, how does it solve it, and the delivery and teaching methods that are used.
Why a SaaC?
Why is there a need for a new learning format?
Although I came up with the denomination and acronym for the Solution-As-A-Course concept in June this year (2024), I started developing the approach in 2018 and matured it over time, understanding what is not working so far, analyze the cause and finding new angles to solve each point:
1 - The absurd education situation in regard to data:
We are surrounded my numbers in our lives, so we’ve all learned calculus in school.
We are surrounded by machines, so we’ve all learned computer literacy.
As undeniable as the above statements: we are surrounded by data, and how absurd as it may sound, data literacy isn’t taught anywhere (apart from dedicated learning paths for experts of course)
Imagine that when you need to create a spreadsheet in Excel, you need to contact an Excel specialist, scope the request, organize meetings with a project team, build a SOW, define milestones and deadlines, …
It sounds absurd, but believe it or not, it is exactly the situation with data literacy today. When people could solve 80% of their data problems by themselves as easily as creating a spreadsheet, we resort for now to two things only:
--- buy tools
--- hire data science teams
2 - Using Tools and AI (both are sort of redundant) isn’t data literacy.
Trainings focused on using tools or the plethora of seminars about “prompt engineering” for genAI do not teach anything about data, just how to become an operator of black box systems.
It is needed of course, but it isn’t data literacy.
Don’t get me wrong, tools and experts are badly needed, but it is not the point here. The point is to make everyone more self-sufficient, make better use of their tools and data expert colleagues, deal with a majority of their data problems by themselves and communicate better.
3 - Data skills shortage:
since this “Excel Engineers”-mindset is the current answer to digitalization, of course there is a shortage of candidates for data science positions. And to be realistic, if we want to continue this way, we will have to wait a few decades before the next generation is ready.
4 - Data Trainings:
The offering out there is impressive, you find so much amazing content that it’s hard to decide which learning path to choose.
But only for the braves: long learning paths over months or years, and although the examples of what we can solve are exciting, there is little hands-on and spot-on programs out there. Like learning a foreign language, if you don’t have a use for it, you will forget all about it.
5 - Cost of developing a custom solution:
a bespoke solution adapted to your industry and the concrete needs of the people in your organization is the ultimate goal. So you can get a tool (that by essence fulfils only part of the requirements) and/or a bespoke program developed specifically for you by engineers. Everyone reading this will know about the costs, the project management journey, the timeframes, the potential lack of adoption, and possible issues when deploying it on the long term.
6 - Cost of AI:
the entry-level subscriptions are free for most AI tools, but soon enough, you end up spending quite a lot. Very often it will be cent by cent, but before you know it, the bills will add on.
The need for Data Literacy is well established and acknowledged by most, but some questions remain: What for concretely? What should be taught exactly, and how? How much knowledge is too little or too much?
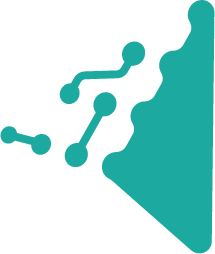
With this in mind, I developed the SolutionAsACourse concept that delivers two things:
-
A working solution to a real problem experienced by real people in an organization.
-
Data education, for people to not only learn the foundations of data science, the processes, but also really own the solution, maintain it, expand it, and create new one
Here’s the official checklist of all requirements for a SaaC:
What topics should a SaaC address?
People first:
in each business function (legal, marketing, sales, HR, R&D, …), people have a set of data-related tasks that takes most of their time, this is what you want to solve, tailoring your course for specific people.
Real data problem:
for an all-audience course, it will be topic experienced by everybody in a business function. Facilitating contract review, automating entire workflows, generating reports, dashboards and presentations, …
What qualifies as a data problem:
generally long and/or repetitive tasks are the best candidates. There can be other cases, such as establishing a pricing policy, which is done one time but with regular checks to adapt it over time. *
* Many startups for instance don’t spend much time on this and it needs a data-safe approach that checks expectations of all parties: investors, board, top management, clients, sales staff, marketing, …
Who should identify the topic of your SaaC:
This can be the most difficult part, since the answers come from the very people you will deliver the course/solution to. You don’t know in details what they do every minute of every day, and they don’t know about data yet, what is possible, how to identify or formulate what takes them time.
So you have to use some sort of Maieutic (the Socratic midwifery approach to help people formulate things). I came up with a few possible questions that could be:
--- “What takes you time and won’t come in your annual performance review?”
--- “What professional tasks do you sometimes spend your evenings or weekends on?”
--- “Which emails have been sitting in your inbox for more than 2 weeks?”
--- “Check your clipboard history, what’s in there?” (i.e. how much copy/paste do you do every day)
--- “Are there any repetitive emails you send every day?”
Selecting the right topic(s):
focus first on what takes the most time per week or month, then on what is the easiest to solve
Quantify a real ROI, how a SaaC helps people save time:
We need to measure or estimate: how long does the task take today? (per day, week, month, …) how long does the solution take? (x seconds, y minutes)
Then you can confidently say something like this:
“what takes 5 hours/week to 3 employees, can be run in less than 2 minutes per employee/week instead, with free technologies”.
The solution has to work:
the solution must be prepared and tested up-front by the instructor, it has to work flawlessly.
In which ways should a SaaC solve the problems?
It must save time:
our ROI point again, hours-long or days-long tasks must be cut down to a few second or minutes only
With free, open-source data science languages:
the technologies used must be free and not captive to a vendor. Learners will just need to install the R language or Python (the most used open source language in data science) and an IDE, the interface to interact with the language. Both are free and safe to install, with unlimited online resources, tutorials and how-to’s.
Out-of-the-sandbox:
this is not a fun science project on the side, it’s a real-life solution, so the solutions will be deployed directly on the machine of the users.
Use real data:
for all-audiences courses use data as close as possible to reality (contracts, regulations, …). For bespoke courses, use data of the organization you develop the courses for.
Replicable:
the solution must be replicable to similar applications and provide for different data input format.
Empower people:
learners need to have the tools and knowledge to maintain and expand the solution independently.
COURSE FORMAT
-
What are the format requirements for a SaaC?
Quick learning cycles:
keep the courses under 8 hours and not just in one chunk, 2 hours per week over a one-month period is the ideal format. When people need more learning, it is possible to deliver some more in-depth knowledge around other data topics.
Bring solid foundations:
make sure you cover the core elements such as data structures, object-oriented concept, … either at the beginning or spread across the entire course
It’s meant for beginners:
and beginners who don’t have the time or patience to go through a formal data science learning path, and yet, for beginners who badly need this data knowledge. The course should constantly show quick wins, while building little by little a mental model that they can expand.
Introduction to the SaaC:
since you teach a new mental model, show a mind map of the course content, not a linear list of items. Remember that the arrows linking the bubbles must contain precise and comprehensible action verbs.
The “Country Parson’s Formula”:
First, start the course by showing what you will solve (bubble on the right with the final output/objective), then the starting data (bubble on the left, which data do you start with), and the main steps in plain English in between. Make it a simple to follow project design.
Second, deliver the SaaC, the building blocks towards the big solution.
Third, as a conclusion, show the mind map again, with a progressive reveal, showing the middle elements in greater details, what we’ve learned, where to find resources.
Start from a blank page: don’t show the full solution and explain each line, but rather write each new line from scratch on a blank page, this is how people will proceed when they create their own solution in the future.
Simplicity:
spend time crafting clear explanations of new concepts you present. Some can be tricky and empiricism may be your friend here. After explaining how you just solved a problem, generalize it to the concept. A series of formative assessments will be needed after this.
No or very little homework:
solving tasks at home, struggling then finding answers, … all are very good learning methods, but a SaaC is not a formal data science course. You can give a taste of it, but you want to provide a quick and painless learning experience.
Fun:
make it as much fun as you can, and if not, solutions that work and problems or errors fixed under a minute should be enticing enough.
Engaging:
with code along, group exercises, formative assessments to diagnose any potential misunderstanding (every 15 minutes or every time that up to 3 new concepts have been introduced), …
Teach how to exploit resources:
how to search for answers, validate which ones are valid, get cheat sheets
Don’t record the course:
this is not a strict pre-requisite, more an advice. If your teaching material is clear enough, people won’t need to watch the course again, this would be a loss of time. More importantly, I realized from experience that a lot of people engage less when they know that they are recorded, or will be reluctant to share their screens to show solutions to their assignments.
WRAP UP
When digitalization, the data world and AI have completely changed our world, our education system stayed roughly the same as in the 20th century.
As a result, we are decades behind when it comes to data education, our reliance on tools and genAI increases the huge gap between data experts and non-experts.
And yet, data literacy is not only possible, but very much needed for any professional or citizen to thrive in this new age.
Catching up with these decades-long neglecting of data education is possible and initiatives such as Solution-As-A-Course are vital.
Our approach delivers two things at the same time to professionals without any formal data science experience:
-
A working solution to their real data problems
-
Data Science education centered around creating the solution
What does it bring to companies:
Very low budget and time resources (in comparison to buying, implementing and boosting adoption of new tools for instance)
Huge impact on individuals and the organization in general
Each repetitive or hours-long task can be cut down to a fraction of the usual time.
It isn’t meant to replace, but rather supplement greatly to purchased tech and data expert teams
Applications are limitless, we help you find which ones will be the most impactful for the people in your organization.
With this new concept, learners will be amazed what they could learn and implement in such a short time, the journey will sound fun and easy. And with their new data science mental model, they will be able to replicate it many new topics.